In the past decade, 3D data acquisition and data processing have increasingly become feasible and important in the domain of photogrammetry and remote sensing. Hence, the automatic semantic segmentation of the huge amount of acquired geospatial data is a crucial task. The semantic segmentation assigns class labels such as buildings, vegetation, impervious surfaces, etc. to each entity.
Images and point clouds are fundamental data representations for geospatial data, particularly in urban mapping applications. Nowadays, joint photogrammetric and LiDAR acquisition is state of the art for airborne systems (hybrid acquisition). Textured 3D meshes intrinsically integrate both data sources by wiring the point cloud and texturing the surface elements with high-resolution imagery (hybrid data storage). Furthermore, meshes facilitate profound geometric data fusion when LiDAR data and aerial imagery have been jointly oriented (hybrid adjustment).
Concerning the recent hybridization trend, from our point of view, enhancing 3D point clouds to textured meshes may replace unstructured point clouds as default representation for urban scenes in the future. For these reasons, we strive for semantic segmentation of textured urban meshes by integrating information from both modalities (hybrid semantics).
We develop a holistic geometry-driven association of entities across modalities aiming at joint multi-modal semantic scene analysis (see Figure 1). We choose the mesh as the core representation due to its integrative character. The association explicitly establishes connections across entities of different modalities. In particular, it recovers the explicit one-to-many relationship between a face and points, which is not encoded in hybridly generated meshes (yet).
The established connections allow propagating information across the modalities imagery, point cloud, and mesh as depicted in Figure 1. The propagated information may enclose manually assigned labels, automatically generated predictions, or modality-specific and handcrafted attributes.
The label transfer enables the consistent semantic segmentation of these modalities while reducing the manual labeling effort. Besides, it facilitates the training of dedicated machine learning algorithms such as CNNs in image space and PointNet++ on point clouds. At the same time, the feature transfer enables the enhancement of per-entity descriptors with available features of other representations (multi-modality).
Figure 2 shows exemplary results for two dedicated feature vector compositions demonstrating the superiority of the multi-modal information integration for the ISPRS benchmark data set Hessigheim3D (H3D).
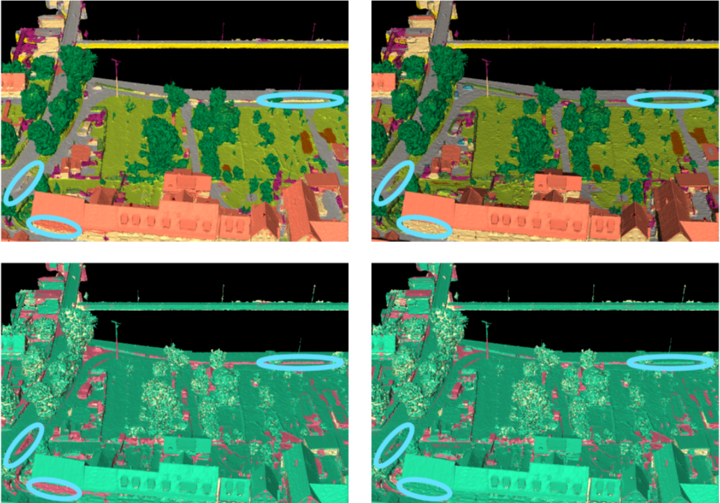
References
Laupheimer, D. (2022), On the Information Transfer Between Imagery, Point Clouds, and Meshes for Multi-Modal Semantics Utilizing Geospatial Data. Dissertation, University of Stuttgart, 2022, 149 S., http://dx.doi.org/10.18419/opus-12668.
Kurzfassung, Runder Tisch GIS
https://www.rundertischgis.de/wp-content/uploads/2024/03/Abstractsammlung-2024.pdf#page=19
Laupheimer, D. & Haala, N.: Juggling With Representations: On the Information Transfer Between Imagery, Point Clouds, and Meshes for Multi-Modal Semantics. ISPRS Journal of Photogrammetry and Remote Sensing, 176 (2021), 55-68, 2021.
DOI: 10.1016/j.isprsjprs.2021.03.007
Laupheimer, D.; Shams Eddin, M. H. & Haala, N.: On the Association of LiDAR Point Clouds and Textured Meshes for Multi-Modal Semantic Segmentation. ISPRS Ann. Photogramm. Remote Sens. Spatial Inf. Sci., V-2-2020, 509–516, 2020.
DOI: 10.5194/isprs-annals-V-2-2020-509-2020
Laupheimer, D., Shams Eddin, M. H., and Haala, N.: The Importance of Radiometric Feature Quality for Semantic Mesh Segmentation, DGPF annual conference, Stuttgart, Germany. Publikationen der DGPF, Band 29, 2020. https://www.dgpf.de/src/tagung/jt2020/proceedings/proceedings/papers/27_DGPF2020_Laupheimer_et_al.pdf
Tutzauer, P., Laupheimer, D., and Haala, N.: Semantic Urban Mesh Enhancement Utilizing A Hybrid Model, ISPRS Ann. Photogramm. Remote Sens. Spatial Inf. Sci., IV-2/W7, 175–182, 2019.
DOI: 10.5194/isprs-annals-IV-2-W7-175-2019
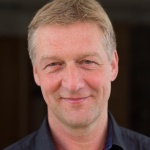
Norbert Haala
apl. Prof. Dr.-Ing.Deputy Director