3D data acquisition and data processing has increasingly become feasible and important in the domain of photogrammetry and remote sensing. Common representations for 3D data are point clouds, volumetric representations, projected views (i.e. RGB-D images or renderings), and meshes.
Textured real-world meshes as generated from airborne LiDAR and airborne oblique UAV imagery provide explicit adjacency information and high-resolution texturing.
Figure 1 shows textured meshes as generated from dense image matching (DIM) only (left) and as derived from DIM and LiDAR data (right) in wireframe fashion.
Meanwhile, textured meshes are a standard representation for the visualization of urban areas.
However, their classification to semantic classes such as buildings, vegetation, impervious surfaces, roads, etc. remains an open research issue.
Current work aims at the integration of complementary airborne LiDAR and aerial image data for an improved semantic segmentation of meshes in the first place.
The attached class labels can also be transferred to point clouds (cf. Figure 2).
Future work will deal with the linking of faces and imagery, and, hence, will extend the existing LiDAR mesh connection to image space.
References
Laupheimer, D., Tutzauer, P., Haala, N. & Spicker, M. (2018) Neural Networks for the Classification of Building-use from Street-View Imagery, ISPRS Ann. Photogramm. Remote Sens. Spatial Inf. Sci., IV-2, 177-184, 2018
DOI: https://doi.org/10.5194/isprs-annals-IV-2-177-2018
Laupheimer, D., Shams Eddin, M. H., and Haala, N.: The Importance of Radiometric Feature Quality for Semantic Mesh Segmentation, DGPF annual conference, Stuttgart, Germany. Publikationen der DGPF, Band 29, 2020.
Tutzauer, P. & Haala, N. [2017] Processing of Crawled Urban Imagery for Building Use Classification. Int. Arch. Photogramm. Remote Sens. Spatial Inf. Sci., XLII-1/W1, 143-149.
DOI: 10.5194/isprs-archives-XLII-1-W1-143-2017
Tutzauer, P., Laupheimer, D., and Haala, N.: SEMANTIC URBAN MESH ENHANCEMENT UTILIZING A HYBRID MODEL, ISPRS Ann. Photogramm. Remote Sens. Spatial Inf. Sci., IV-2/W7, 175–182, 2019.
DOI: 10.5194/isprs-annals-IV-2-W7-175-2019
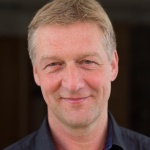
Norbert Haala
apl. Prof. Dr.-Ing.Deputy Director