Urban Data is available in a variety of representations, ranging from street-level imagery over coarse building models to dense point clouds and colored meshes. However, essential semantic data like building use categories is often not available. This project addresses the issue by proposing a pipeline to use crawled urban imagery and link it with ground truth cadastral data as an input for automatic building use classification. The aim is to extract city-relevant semantic information from Street View (SV) imagery or other publicly available urban data sources.
The example from our Street View crawler with red markers denoting crawled positions depicts a quasi-frontal shot of facades as available from this data source. Another valuable source of information is Volunteered Geographic Information (VGI). As an example, within Mapillary individual users can produce street-view scenes under Creative Common license. In general, the more training data, the better classification will generalize and in turn provide better results. While imagery is usually available to a sufficient extent, the main bottleneck is the amount of ground truth. In our scenario this is provided from a digital city base map as it is made available by some as part of open data initiatives.
The figure exemplary shows façade imagery with predicted class and with associated percentage of confidence. The overlaid Class-Activation-Maps (CAM) highlighting image areas which triggered activation of the network. As an example, the class Under Construction is predicted with a probability of 100% due to the scaffolds and the crane defining regions with the highest activations.
Contact
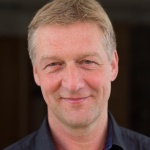
Norbert Haala
apl. Prof. Dr.-Ing.Deputy Director