Steffen Merseburg
Sentinel 1 SAR images towards their application in civil Search and Rescue
Duration: 6 months
Completition: April 2024
Supervisor and Examiner: Prof. Dr.-Ing. Uwe Sörgel
Introduction
The Mediterranean Sea, often referred to as the deadliest border in the world, witnesses the tragic loss of thousands of lives each year. In response to this ongoing humanitarian crisis, NGOs have deployed ships and airplanes for Search and Rescue operations. Satellite imagery could complement these efforts, as it gives an excellent overview of the situation but lacks real-time capabilities and detail. However, the European border and security agency Frontex is already using satellite images to spot people in distress together with the EMSA, proving the effectiveness of satellite images in the context of Search and Rescue [1]-[2].
Sentinel-1 images are ideal for civil actors because they are distributed free of charge within three hours of acquisition. However, detecting tiny boats refugees use is especially challenging because of the coarse spatial resolution of these images. The expected detection rate for 25-34-meter ships in Sentinel 1 images is 90% [3]. However, this theoretical value hinges on the well-known Constant False Alarm Rate detector dating back to 1966 [4]. Many studies still use the CFAR Detector, which gives reasonable results for low computation costs. With the rise of machine learning, we can find models that perform better and push the ship size detectability further. The problem of detecting particular small targets in large scenes has been of interest in machine learning, especially in the remote sensing community [5]-[7]. Specialized architectures were proposed to tackle these problems. This study explores using CNNs to detect tiny ships in Sentinel-1 images.
Methodology
The approach of this study can be split into four phases:
- Dataset gathering
- Metric
- Baseline Algorithms
- CNN Development
- Analysis of best Algorithms
Dataset
The dataset is gathered with the help of Automatic Identification System (AIS) Data in the Mediterranean Sea. The position timestamps are interpolated to the acquisition time of the Sentinel-1 images. As the AIS position is imprecise a bounding box can not be drawn directly from this data. Therefore, a classification dataset is built with 256-pixel cut-outs, randomly shifted by only 53 pixels to ensure that the boat is still in the image. Another cut-out with a similar sea state is made to get a perfectly balanced dataset. The AIS data and the CFAR algorithm ensured that no boat was present in the sea cut-out. The scalable approach lets us create a dataset of 6192 cut-outs from 1080 different Sentinel-1 images. For each cut-out, metadata about the ships, the weather conditions, and the Sentinel-1 image metadata is collected for deeper analysis.
Metric
In the context of Search and Rescue, it may be more important not to miss a potential case than to have a false alarm. To train the models correctly, a metric is required that reflects this and punishes false positives less than false negatives. Furthermore, trivial cases of always bedding on true or false should also be punished. Unfortunately, there is no metric with this property, so a new Metric, FM3, was designed.
Baseline Algorithms
For a baseline, a series of traditional algorithms using filtering techniques such as Polarimetric Whitening Filter or Depolarization Ratios in combination with the CFAR algorithm are used. Furthermore, a series of lightweight machine learning models available from PyTorch were used, all with less than 12 million trainable parameters.
CNN Development
A series of architectures based on the RegNetY and the TinyNet with different micro- and macro-level variations were designed to improve detectability further. Among the micro-level design choices are dilated convolutions to increase the receptive field, max pooling operations instead of strided convolution for downsampling operations, complex-valued inputs and 3D channel-wise convolution.
Analysis of the models
The models are trained 30 times independently with slight variations in the hyperparameters to measure how well they converge and what performance is achievable. The best models are further analyzed by looking at what external factors, like weather and ship size, influence the performance. To visually verify that the machine learning model is detecting the presence of a boat and not specious correlations, Grad-CAM ++ analysis is made. The models are tested on real Search and Rescue cases reported close to the acquisition of a Sentinel-1 image to test if the transfer from boats with AIS signals to refugee boats works. Lastly, the execution speed is evaluated on a complete Sentinel-1 image.
Results and Discussion
The Baseline algorithms only achieved a relatively low FM3 Score of 0.7, with the traditional MMSE-PWF filtering and CFAR detection reaching the highest score, followed by three machine learning models, the ResNet18, RegNetY, and DenseNet 121 as can be seen in Figure 1.
The best technique for the newly developed CNNs was the yBlock, which uses a residual structure and squeeze excitation according to Figure 2. The most noticeable improvement probably came from using the max pooling operation for downsampling operations. For global pooling operations, max pooling was again superior to average pooling, but not using global pooling produced the best results. The dilated convolutions produced promising results with a much higher median score over the different training trials.
The most noticeable external factors were the boat's length and wave height. The influence of incident angle was surprisingly low, which is likely explainable given the use of the CFAR algorithm in the making of this dataset.
The Grad-CAM ++ analysis clearly shows that the proposed model detects the ship's presence, even though traditional algorithms and SAR experts find it challenging to spot the boat in the image.
Unfortunately, the good results can not yet be verified in actual cases. The analysis of three past cases is either inconclusive or only conclusive when different detectors are combined.
The proposed model proved to be much faster, running at 2586 FPS on a commercial-grade GPU, compared to the traditional algorithm, which runs at 96 FPS on a commercial-grade CPU.
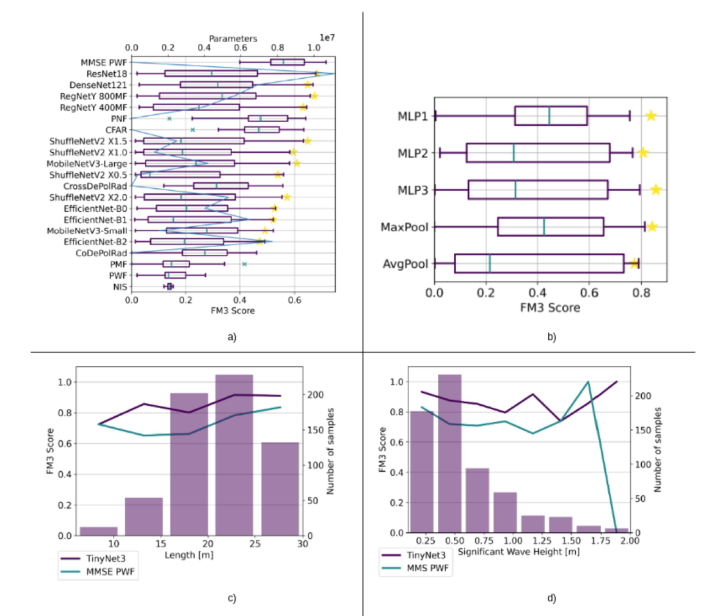
Conclusion and further direction
In this thesis, we have achieved a milestone by successfully pushing the boundaries of tiny ship detectability using a novel Sentinel-1 dataset in combination with new CNN designs. Two novel design choices are introduced, which show noticeable improvements for CNNs in tiny object detection. We then explored its application in civil Search and Rescue by analyzing real cases and a theoretical monitoring application. This study, which encompasses various aspects from model design to limitations and possible applications, opens up numerous avenues for further exploration.
The novel dataset approach led to a few biases, which could be addressed by lowering the CFAR threshold and allowing more false labels in the training.
Interestingly, the approaches driven by the traditional models, namely complex-valued inputs and 3D channel-wise convolution, did not perform as well as expected. This might be because of the methods' lower maturity, which needs to be addressed further.
On the other hand, increasing the receptive field and using max pooling operations led to a good performance of a lightweight classification network that outperformed state-of-the-art results. However, the model showed too many false alarms on the test images, and further research needs to be done before Seentinel-1 images can be used in the context of civil Search and Rescue.
References
[1] |
Copernicus Maritime Surveillance. Use Case - Maritime Safety, en-GB. [Online]. Available: https://www.emsa.europa.eu/copernicus/cms- cases/item/5139- copernicus- maritime- surveillance- use-case-maritime-safety.html (visited on 04/02/2024). |
[2] |
Dokumente des 2. Workshops über Forschung und Entwicklung für die Entwicklung des CopernicusEU-Sicherheitsdienstes vom 12.12.2023, de. [Online]. Available: https://fragdenstaat.de/anfrage/dokumente-des-2-workshops-ueber-forschung- und-entwicklung-fuer-die-entwicklung-des-copernicuseu-sicherheitsdienstes-vom-12-12-2023-1/ (visited on 04/01/2024). |
[3] |
R. Torres, P. Snoeij, D. Geudtner, et al., “GMES Sentinel-1 mission,” en, Remote Sensing of Environment, vol. 120, pp. 9–24, May 2012, issn: 00344257. doi: 10.1016/j.rse.2011.05.028. [Online]. Available: https://linkinghub.elsevier.com/retrieve/pii/S0034425712000600 (visited on 04/07/2024). |
[4] |
H. Finn, “Adaptive detection in clutter,” in Fifth Symposium on Adaptive Processes, USA: IEEE, Oct. 1966, pp. 562–567. doi: 10.1109/SAP.1966. 271149. [Online]. Available: http://ieeexplore.ieee.org/document/4043676/ (visited on 03/13/2024). |
[5] |
N. Pawlowski, S. Bhooshan, N. Ballas, F. Ciompi, B. Glocker, and M. Drozdzal, “Needles in Haystacks: On Classifying Tiny Objects in Large Images,” 2019, Publisher: [object Object] Version Number: 2. doi: 10. 48550/ARXIV.1908.06037. [Online]. Available: https://arxiv.org/abs/1908.06037 (visited on 03/05/2024). |
[6] |
J. Pang, C. Li, J. Shi, Z. Xu, and H. Feng, “R²-CNN: Fast Tiny Object Detection in Large-Scale Remote Sensing Images,” IEEE Transactions on Geoscience and Remote Sensing, vol. 57, no. 8, pp. 5512–5524, Aug. 2019, issn: 0196-2892, 1558-0644. doi: 10.1109/TGRS.2019.2899955. [Online]. Available: https://ieeexplore.ieee.org/document/8672899/ (visited on 03/05/2024). |
[7] |
T. Zhang, X. Zhang, X. Ke, et al., “LS-SSDD-v1.0: A Deep Learning Dataset Dedicated to Small Ship Detection from Large-Scale Sentinel-1 SAR Images,” Remote Sensing, vol. 12, no. 18, p. 2997, 2020. doi:10.3390/rs12182997. |
Ansprechpartner
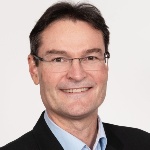
Uwe Sörgel
Prof. Dr.-Ing.Institutsleiter, Fachstudienberater