Dilara Elif Yildiz
Development of a Multi-Cam-SLAM System for Industrial Environments
Duration: 6 months
Completion: July 2024
Supervisor: M.Sc. Vincent Reß
Examiner: Prof. Dr.-Ing. Norbert Haala
Introduction
Autonomous Systems are becoming increasingly prevalent in today's manufacturing industries due to their enhanced capabilities in productivity, precision, and safety. To effectively interact with their complex industrial environments, these systems must accurately determine their positions. Conventional VSLAM algorithms, using monocular or stereo cams, face challenges in determining scale and varying lighting conditions. While they demonstrate acceptable results in their backend estimation through global bundle adjustment, they struggle to provide precise poses during robot movement based solely on frontend estimations.
Emerging solutions include multi-camera setups and sensor fusion, which offer a broader view and rich information of the environment. However, existing multi-cam systems require cameras with significant overlapping and synchronization, which is often not feasible in practice. This thesis proposes a visual-inertial multi-camera-SLAM-system that can operate with non-overlapping and asynchronous cameras. Building on DROID-SLAMs framework (Teed & Deng, 2021), it combines graph and deep learning-based approaches with an error state extended kalman filter to enhance trajectory estimation in the frontend.
System Architecture
Figure 1 illustrates the high-level architecture of the developed system, operating with three non-overlapping, asynchronous cameras, but is scalable to any number of cameras.
- Local Pose Graphs: For each camera an independent tracking process starts, constructing a local pose graph from selected keyframes. These tracking processes provide preliminary pose estimates for each camera.
- Error State Extended Kalman Filter (ESEKF): The core of the system is a central ESEKF that uses IMU data for preliminary pose estimation (prediction) and refines it with the incoming camera poses from the tracking processes (correction). The filter is based on the ESEKF code of Bashmakov (Bashmakov, 2024).
- Pose Manager: A pose manager coordinates communication between the ESEKF and the tracking processes, collecting latest poses and forwarding them to the ESEKF. It also sends corrected poses back to the local pose graphs to ensure consistency.
Results
The performance of the system is tested on a trajectory within an industrial factory hall and compared to the ground truth obtained by tachymeter measurements.
Figure 2 visualizes the results of the multi-cam SLAM algorithm tested with two cameras (front and rear cam). Despite the communication between the ESEKF and the tracking processes, the cameras drift apart. The ESEKF correction follows a zigzag pattern and jumps back and forth between the pose estimates of both cameras.
Challenges and improvements
The tests confirm the system's functionality and its ability to integrate asynchronous, non-overlapping image sources with IMU data into an estimate. However, the experimental results showed only marginal improvements. The main reason for this phenomenon is a limited influence of the ESEKF. The initialization of frame graphs overwrites the initial ESEKF corrections, reducing its influence early in the trajectory estimation. The ESEKF's impact on local pose graphs remains minimal, causing camera trajectories to drift and resulting in a suboptimal zigzag pattern in the joint ESEKF estimate.
To improve the developed algorithm, the following measures are proposed:
- Strengthening ESEKF influence: Modify the initialization to start the filtering process later and ensure consistency between the filter and local tracking processes. Exchanging multiple poses per time step could also enhance ESEKF's influence on local pose graphs.
- Linking camera-specific pose graphs: The parallel pose graphs require significant GPU resources, limiting scalability. Linking local graphs during frontend tracking using inter-camera factors that include IMU estimates could enhance communication between graphs and reduce GPU resource requirements, allowing for a more compact graph optimization and consistency between the camera tracking processes.
References (Selection)
Bashmakov, P. (2024, Mai). Lidar odometry smoothing using ES EKF and KissICP for Ouster sensors with IMUs. https://capsulesbot.com/blog/2024/02/05/esekf-smoothing-ouster-lidar-with-imu-using-kiss.html
Cadena, C., Carlone, L., Carrillo, H., Latif, Y., Scaramuzza, D., Neira, J., Reid, I., & Leonard, J. J. (2016). Past, Present, and Future of Simultaneous Localization And Mapping: Towards the Robust-Perception Age. IEEE Transactions on Robotics, 32(6), 1309–1332. https://doi.org/10.1109/TRO.2016.2624754
Solà, J. (2017). Quaternion kinematics for the error-state Kalman filter (arXiv:1711.02508). arXiv. https://doi.org/10.48550/arXiv.1711.02508
Teed, Z., & Deng, J. (2021, August 24). DROID-SLAM: Deep Visual SLAM for Monocular, Stereo, and RGB-D Cameras. Neural Information Processing Systems. https://www.semanticscholar.org/paper/DROID-SLAM%3A-Deep-Visual-SLAM-for-Monocular%2C-Stereo%2C-Teed-Deng/67515d1f7df144683b059e684da7974e40aeaca1
Thrun, S., Burgard, W., & Fox, D. (2005). Probabilistic Robotics (Illustrated edition). The MIT Press.
Ansprechpartner
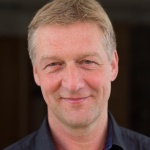
Norbert Haala
apl. Prof. Dr.-Ing.Stellvertretender Institutsleiter