Jiaxin Liu
Analysis, Comparison, and Enhancement of NeRF and 3D Gaussian Splatting-based Visual RGB-D SLAM
Duration: 6 months
Completition: June 2024
Supervisor: M.Sc. Wei Zhang
Examiner: Prof. Dr.-Ing. Norbert Haala
Introduction
Simultaneous localization and mapping (SLAM) is the computational problem of constructing, updating, and optimizing a map of an unknown environment while simultaneously keeping track of the location within it. Traditional visual SLAM methods achieve real-time mapping and tracking but only generate sparse maps, which lack the detail required for advanced appli- cations such as navigation and 3D reconstruction. Dense visual SLAM methods aim to recon- struct detailed 3D maps and can be divided into view-centric and world-centric approaches, each with their own advantages and limitations. Recently, radiance field representations, such as Neural radiance field (Mildenhall et al., 2020) and 3D Gaussian Splatting (Kerbl et al., 2023), have become popular for detailed scene reconstruction.
This work explores the integration of 3D Gaussian Splatting with SLAM, leveraging its ability to render high-quality 3D scenes with a fast differentiable rasterizer. We conducted a compre- hensive study on NeRF-based SLAM, transitioning to 3DGS, to assess its potential benefits and feasibility. We then developed a custom 3DGS-based RGB-D SLAM framework, refining its integration to enhance scene reconstruction and camera tracking capabilities. Extensive exper- iments were conducted to compare our framework with NeRF-based and other 3DGS-based SLAM methods across various datasets and scenarios. Our evaluation highlights the strengths and weaknesses of our 3DGS SLAM framework, providing insights into its potential and guid- ing future improvements.
The system overview of our 3DGS-based SLAM framework is summarized in Fig. 1. The frame- work begins with an initial map consisting of a set of 3D Gaussians based on the first RGB-D frame t0. The Gaussians will be efficiently initialized with the help of the depth image and then iteratively optimized.
Every new frame t will be tracked and checked for keyframe registration. By employing a loss function, the loss is backpropagated to iteratively update the camera poses. Special attention should be paid to the fact that DROID-SLAM is used as an additional option to assist the cam- era tracking, providing, in most cases, a fast and accurate localization, which speeds up the optimization process and the convergence of the parameters. keyframe must be detected af- ter tracking to improve efficiency and avoid redundant information and unnecessary memory consumption. The determination of keyframes in our framework is a combination of several benchmarks that will take into account the translation between cameras, the covisibility area, the fraction of the frame already explained by the map, and DROID-SLAM’s keyframe strategy.
Once a keyframe is detected, pixels from the current frame are sampled, and new Gaussians are generated according to a specific strategy. Immediately after, a active map optimization is performed to update the 3D Gaussians. This optimization involves carefully selecting and optimizing a subset of keyframes to prevent catastrophic forgetting and overfitting, which can occur when optimizing only a single frame. All Gaussians within the active map are optimized over a certain number of iterations by defining a mapping loss. To better preserve the geometric density obtained from the depth sensor while controlling the number of 3D Gaussians within a suitable range and reducing computation time, we perform the densification and pruning at relatively sparse intervals during the mapping process. Finally, after completing all camera tracking, a global joint optimization is performed to further refine the camera poses and 3D Gaussians.
Experiment
Our 3DGS-based SLAM framework will be tested on the synthetic dataset Replica (Straub et al., 2019), the real-world dataset TUM-RGBD (Sturm et al., 2012), and our own captured dataset. All the datasets are run on an Ubuntu 22.04 desktop with Intel Core i7-13700K 3.4GHz and a single NVIDIA GeForce RTX 4080 Super.
Tracking Performance
Absolute Trajectory Error (ATE) is used to measure the tracking performance. We compared our method with the NeRF-based methods, as well as the GS-based methods, across three scenes in the Replica dataset and two scenes in the TUM RGB-D dataset. Specifically, since our method integrates Droid-SLAM, we also compared its performance. The table 1 shows the ATE RMSE for different methods across all scenes.
It demonstrates that the combination of 3DGS and DROID-SLAM is highly effective, consis- tently delivering superior performance across nearly all scenarios. In fact, DROID-SLAM can run efficiently in real-time at around 30 FPS, though it sacrifices performance for efficiency. On the other hand, 3DGS-based SplaTAM and MonoGS achieve excellent localization perfor- mance, but run significantly less efficiently, between 0.3 and 0.5 FPS. This reduced efficiency is primarily due to the large number of iterations required to optimize camera poses and Gaussian parameters. As previously mentioned, our framework offers the potential for integration with DROID-SLAM during camera initialization. Using the rapidly estimated poses from DROID- SLAM as initial values not only improves efficiency (1 FPS to 2 FPS) and reduces the number of iterations but also enhances accuracy and significantly increases robustness.
Mapping Performance
Three metrics (PSNR, LPIPS, SSIM) were utilized to evaluate the rendering results comprehen- sively for the quantitative analysis of mapping performance. These metrics were calculated by rendering images from all camera viewpoints and comparing them with the ground truth, ensuring a robust and thorough assessment of the rendering accuracy.
Table 2 presents the rendering performance. The experimental results clearly demonstrate that the performance of the two NeRF-based SLAM frameworks is significantly lower than the 3DGS-based SLAM framework. Gaussian Splatting exhibits outstanding performance in map- ping and novel view rendering. It can also be seen that the combination of DROID-SLAM and 3DGS achieves impressive results, performing satisfactorily in all aspects. This is especially evident in the dataset we captured. However, it should be noted that the numerous parame- ters that control the entire SLAM framework are highly complex. These parameters range from the weights of different losses in the loss function, the number of iterations for tracking and mapping, keyframe selection parameters, and Gaussian-related parameters, etc.
Conclusion
In this paper, we have undertaken a comprehensive exploration and development in the field of dense visual RGB-D SLAM utilizing NeRF and 3D Gaussian Splatting. We focus on the development of an innovative 3DGS-based RGB-D SLAM framework. Our method has demon- strated superior performance in most cases compared to existing methods. However, although our method enhances many details and improves performance, 3DGS-based SLAM still faces several problems:
- Low Efficiency: The computational demands of 3DGS-based SLAM can lead to lower efficiency, making real-time processing challenging.
- High Hardware Requirements: The advanced algorithms and processing capabilities re- quired by 3DGS-based SLAM necessitate high-end hardware, which may not be accessi- ble for all applications.
- Suboptimal Performance in Large Scenes: 3DGS-based SLAM struggles with effectively reconstructing large scenes, often resulting in less detailed representations.
- High Multi-View Input Requirements: The need for input images from multiple view- points to optimize the scene makes the process more complex and resource-intensive.
Further research is needed to address the remaining challenges. Despite these challenges, the development potential of 3DGS in SLAM is huge and promising.
Ansprechpartner
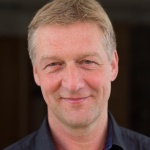
Norbert Haala
apl. Prof. Dr.-Ing.Stellvertretender Institutsleiter