Peiwei Pan
Dense Collaborative Mapping with Deep Visual SLAM Method
Duration: 6 months
Completition: May 2024
Supervisor: M.Sc. Wei Zhang
Examiner: Prof. Dr.-Ing. Norbert Haala
Introduction
The creation of highly accurate and collaborative mapping algorithms is crucial for the progress of SLAM technology, as it greatly improve the efficiency of building detailed maps. In the area of mapping based on single moving trajectory, DROID-SLAM (Differentiable Recurrent Optimization-Inspired Design) by Teed and Deng (2021) stands out as an innovative method based on deep learning, providing a visual-only solution that works with various types of camera, such as monocular, stereo, and RGB-D. Its ability to create maps with excellent accuracy makes it superior to well-known methods like ORB-SLAM3 by Campos et al. (2021). Despite its impressive individual mapping performance, DROID-SLAM does not account for scenarios involving multi-session data or the collaborative map creation by multiple agents.
To address this problem, we propose two collaborative map construction algorithms built upon DROID-SLAM. Compared to prior methods that compute explicit relative transformations for loop closures, our algorithm leverages the power of deep learning-based bundle adjustment, using dense per-pixel correspondence, to merge into a globally consistent state. These algo- rithms have been thoroughly tested with stereo and RGB-D models. we validated the effec- tiveness of our proposed algorithms on both public and self-collected datasets, showing higher accuracy than prior methods. By leveraging the strengths of DROID-SLAM while addressing its limitations with our novel algorithms, we extend the application scenarios of this method and provide a new way of thinking about collaborative mapping.
In Figure 1, the stages of the collaborative map building process are depicted, starting with the extraction of keyframes from image sequences (Upper left corner of Figure 1). This step is crucial as it significantly reduces the data volume for collaborative mapping while ensuring its quality, by focusing solely on keyframes. Leveraging the considerable advantages of DROID- SLAM’s use of deep learning-based optical flow for keyframe selection, we can minimize the keyframes that require processing to the greatest extent.
After extracting keyframes, using Prim’s algorithm (by Prim (1990)) and FBOW (by Zhang et al. (2010)) algorithm to connect the sub-maps sequentially based on the degree of similar- ity between them. For each two submaps to be aligned together, extracting common viewing within them. Then the merging strategy is determined based on the overlapping ratio be- tween submaps. Specifically, if the overlap exceeds half of their area, the process employs method 1 (Transformation matrix calculation method), as shown in the upper half of the Micro DROID Loop unit in figure 1. This method calculates the pose transformation matrix, which is then applied to all keyframes of the submap to be aligned, aligning it with the other reference submap. If the overlap is less than half, method 2 (Poses direct acquisition method) is applied instead. Illustrated in the lower half of figure 1, this method directly calculates the new poses for the submap to be aligned in the reference coordinate system of reference submap, bypassing the need for a transformation matrix. But this method needs to do the local backend optimisa- tion later for the whole submap to be aligned with new poses. This approach is generally used when the spatial relationship between the submaps is less direct.
After choosing the appropriate method, global backend optimization which is the same as the backend process using DROID-SLAM method in two submaps construction plays a crucial role. This optimization process fine-tunes the aligned map, ensuring that it is both coherent and accurate. It adjusts the poses of two submaps based on the overall structure, reducing errors and discrepancies. The iterative nature of this process means that after each pair of submaps is aligned and optimized, the system checks if there are still unaligned submaps remaining. The procedure repeats, merging submaps and optimizing the global structure, until all have been integrated into a unified map.
Experiment
After describing our proposed collaborative mapping method, we need to conduct experiments to demonstrate the effectiveness of our method. We begin by 1) validating the complementary nature of our two proposed collaborative mapping methods, followed by 2) quantitative evalu- ations of camera pose accuracy using the public EuRoC dataset by (Burri et al. (2016)). We then conclude with 3) the evaluation of the merged dense point cloud map using our self-collected dataset.
- Complementary nature of two methods
The Table 1 shows that while Method 1’s time efficiency stands out in areas with high map overlap (MH01-MH03), its accuracy falls in areas with less overlap (MH04-MH05). Adopting a second method that accounts for both overlapping and non-overlapping ar- eas, although time-consuming due to increased keyframe integration and backend opti- mization, improves robustness and maintains accuracy across all sequences, illustrating a trade-off between accuracy and efficiency in collaborative mapping.
- Camera poses accuracy evaluation
In assessing algorithmic accuracy, we first observe the results of map merging after ap- plying appropriate methods, as shown in Figure 2. Subsequently, we quantitatively evaluated the accuracy of the algorithm. This was done by comparing the Root Mean Square Absolute Trajectory Error (RMS ATE) between the trajectories post-collaborative mapping and the ground-truth trajectories. We also compared these results with vari- ous outstanding algorithms(ORB-SLAM3 by Campos et al. (2021), COVINS by Schmuck et al. (2021), Maplab2.0 by Cramariuc et al. (2022)), as shown in Table 2. Specifically, by relying exclusively on camera inputs, our algorithm demonstrates a universally higher accuracy in tracking and localization throughout the camera’s motion. This not only un- derlines the effectiveness of our approach but also highlights its superiority in scenarios where only camera data is available, proving its robustness and advanced capabilities in visual-based.
3. Dense point cloud accuracy evaluation The third experiment tested the accuracy of a reconstructed dense point cloud using a self-collected dataset and an RGB-D camera to capture images of two areas of a room, divided by a bookshelf as shown in Fig 3. With limited shared views, we used the second collaborative mapping method for reconstruc- tion. We then used a high-accuracy LiDAR integrated with a camera and IMU to create a complete room model. Both point clouds were compared in CloudCompare by aligning them manually and measuring discrepancies. The results, displayed in Fig 3, show that the collaborative mapping point cloud predominantly matched the LiDAR data within a 5 centimeter discrepancy, indicating high alignment and validating the collaborative mapping’s effectiveness in accurate spatial data reconstruction.
Conclusion and Outlook
In this paper, we introduce two collaborative mapping approaches tailored for visual SLAM (DROID-SLAM), especially addressing the lack of collaborative mapping in DROID-SLAM and scenarios with limited common viewing areas by proposing a viable solution. These methods have been rigorously validated through extensive experiments, proving to be both necessary and effective.
Method 1 (Transformation matrix calculation method) is based on the calculation of the trans- formation matrix, this method is very time efficient, but in areas with fewer common viewings there will be a significant drop in the accuracy of the alignment. Method 2 (Poses direct ac- quisition method) is to directly solve for the new poses of the to-be-aligned keyframes, which is ideal for situations where there are fewer common viewings, but the time efficiency will be reduced. Therefore these two approaches are complementary.
Remarkably, even in scenarios reliant solely on camera inputs, our approaches achieve a higher collaborative mapping accuracy compared to other algorithms that integrate multiple sensors. This advancement underscores our methods’ efficiency in leveraging visual data, setting a new benchmark for accuracy in the realm of collaborative visual SLAM.
In the future, we plan to incorporate the two proposed collaborative mapping algorithms into a deep learning framework, aiming for an end-to-end solution. This effort will potentially enhance mapping efficiency, paving the way for advanced autonomous systems and multi- robotics applications.
Bibliography
Burri, M., Nikolic, J., Gohl, P., Schneider, T., Rehder, J., Omari, S., Achtelik, M. W. and Siegwart,(2016), ‘The EuRoC micro aerial vehicle datasets’, The International Journal of Robotics Research 35(10), 1157–1163.
Campos, C., Elvira, R., Rodriguez, J. J. G., Montiel, J. M. M. and Tardos, J. D. (2021), ‘ORB- SLAM3: An accurate open-source library for visual, visual–inertial, and multimap SLAM’, IEEE Transactions on Robotics pp. 1–17.
Cramariuc, A., Bernreiter, L., Tschopp, F., Fehr, M., Reijgwart, V., Nieto, J., Siegwart, R. and Cadena, C. (2022), ‘maplab 2.0–a modular and multi-modal mapping framework’, IEEE Robotics and Automation Letters 8(2), 520–527.
Prim, R. (1990), ‘Shortest connection networks and some generation’, Bell Systems Tech. J. 36, 368–379.
Schmuck, P., Ziegler, T., Karrer, M., Perraudin, J. and Chli, M. (2021), Covins: Visual-inertial slam for centralized collaboration, in ‘2021 IEEE International Symposium on Mixed and Augmented Reality Adjunct (ISMAR-Adjunct)’, IEEE, pp. 171–176.
Teed, Z. and Deng, J. (2021), ‘DROID-SLAM: Deep Visual SLAM for Monocular, Stereo, and RGB-D Cameras’, Advances in neural information processing systems .
Zhang, Y., Jin, R. and Zhou, Z.-H. (2010), ‘Understanding bag-of-words model: a statistical framework’, International journal of machine learning and cybernetics 1, 43–52.
Ansprechpartner
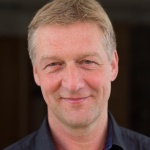
Norbert Haala
apl. Prof. Dr.-Ing.Stellvertretender Institutsleiter
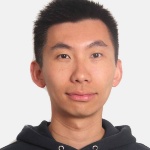
Wei Zhang
M.Sc.Wissenschaftlicher Mitarbeiter