Leonard Buechner
Estimating Canopy Traits from Hyperspectral Satellite Data using Convolutional Neural Networks
Duration: 6 months
Completition: January 2023
Supervisors: : Dr. Nicholas Coops, Dr. Thomas Miraglio, The University of British Columbia, Faculty of Forestry
Examiner: Prof. Dr.-Ing. Norbert Haala
Introduction
Forests are being increasingly stressed under a changing climate, which may impact the functional diversity of forest ecosystems. This can be monitored through vegetation traits, which are variables related to the biochemical and biophysical properties of leaves and canopies (Kattge et al. 2011; Violle et al. 2007). These traits have specific radiative properties that can be measured across landscapes using airborne and spaceborne imaging spectrometers enabling the monitoring of the forest ecosystems’ response to external stressors. However, imaging spectrometers, such as Hyperion (Green, Pavri, and Chrien 2003), have narrow spectral bands, which may result in a poor signal-to-noise ratio and strong collinearity between bands (Rasti et al. 2018). The scarcity of ground truth data coupled with the high dimensionality of hyperspectral images makes it necessary to identify trait retrieval methods that are capable of handling the large spatial variability of spectral features (Berger et al. 2020).
Methods
The mapping of hyperspectral reflectance images to vegetation traits is done using a modified version of the U-within-U-Net (Manifold et al. 2021). Due to the lack of ground truth data, the training process of the model needs to be split into two steps: first, unsupervised training of the spatial part of the model, then transferring the trained weights, and training the spectral part of the model for trait estimation in a supervised way.
Synthetic data are generated using the INFORM model (Atzberger 2000) to associate a reflectance spectrum to vegetation traits. The input parameters to INFORM Xinform include, in addition to others, the traits that are going to be estimated (Ŷtraits) from the HSIs, which allows supervised optimization of the spectral model parameters Figure 1 .
Results
Spatial Reconstruction
The reconstructed images were visually similar to the original Hyperion images. The differences between the original and the reconstruction were the largest near localized features such as the edges of the images, rivers, roads, or snowy mountain peaks. While the SSIM calculated per band across all tiles was slightly lower for wavelengths in the NIR range and showed that the bands in the visible and SWIR ranges were reconstructed more precisely. Overall, it was shown that the reconstruction accuracy was satisfying.
Trait Estimation using Synthetic Data
Models trained (I) only spectrally and (II) combining spatial pre-training with spectral training both resulted in accurate trait estimates reaching over R2 with corresponding NRMSE = 13 % for CBC and Cab . For LAI both models had a R2 score > 0.53 with NRMSE = 15 %, while performances were poorer for PROT (R2 > 0.20 and NRMSE up to 26%), see Figure 2.
Both models performed similarly, and it is likely that the spatial features from pre-training only had a marginal influence on the ability to estimate traits.
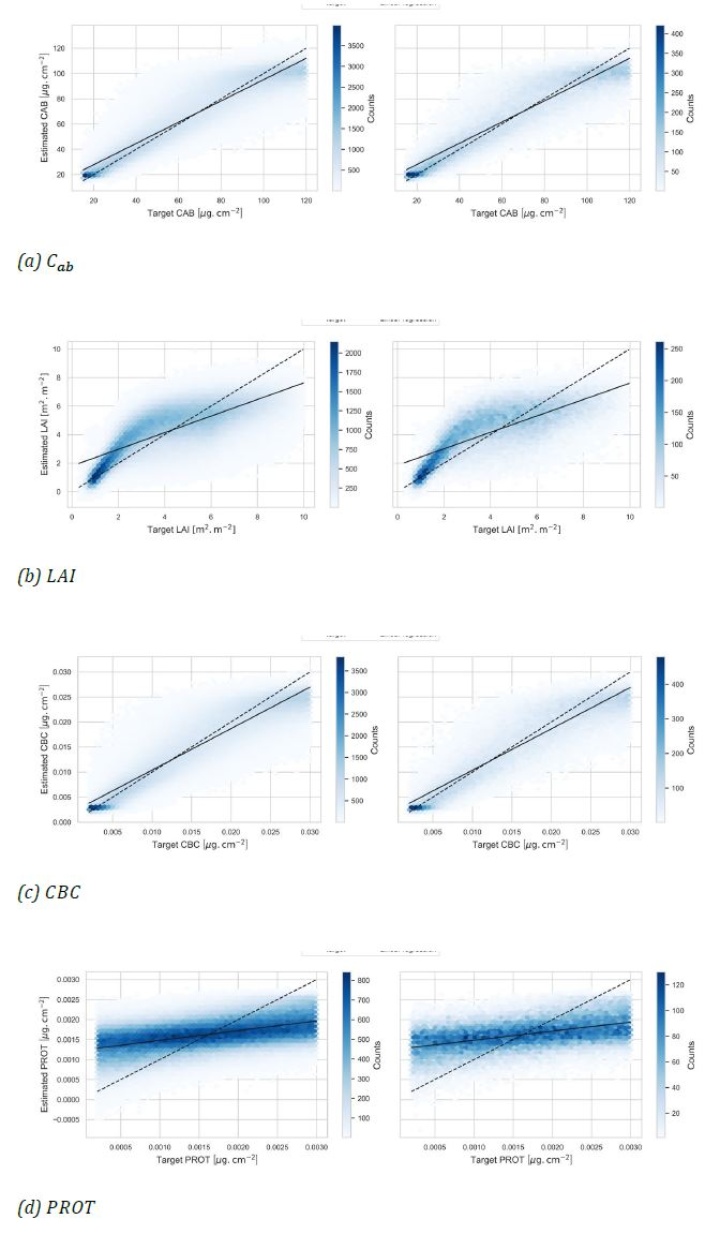
Trait Estimation on the Landscape Scale
While artifacts could be present over tiles containing areas where no reflectance data were available, the trait estimates were overall consistent with visible spatial features within the image for pixels that corresponded to the forested area and had a very high structural similarity (SSIM > 0.95). Comparing the trait estimates between different species showed significantly different patterns for broadleaved and coniferous species for Cab, CBC and PROT, while the estimates were very similar for LAI.
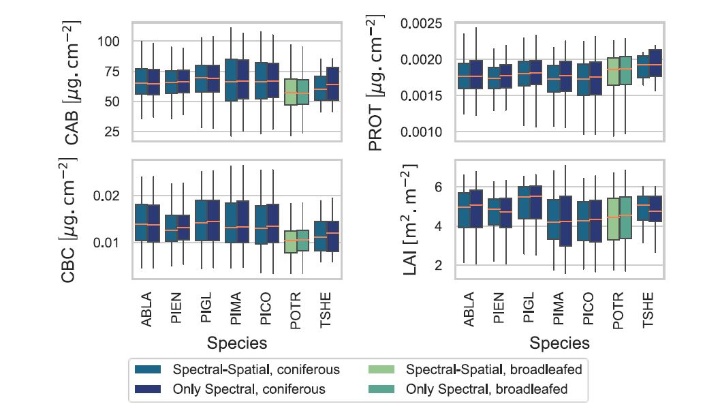
Conclusion
It was shown that multi-trait estimation was possible using a U-within-U-Net trained with synthetic reflectance data. Taking into account both spatial and spectral features yielded encouraging results for the estimation of key functional traits at the landscape scale.
Given the need for accessible estimates of functional traits from remote sensing data, but lacking ground reference data, the use of hybrid models presents a viable option to bridge this data gap.
References (Selection)
Atzberger, Clement. 2000. “Development of an Invertible Forest Reflectance Model: The INFOR-Model,” 11. https://d1wqtxts1xzle7.cloudfront.net/7001326/atzberger_inform-with-cover-page-v2.pdf?Expires=1668115359&Signature=auVLS1Xlo4lQJxD-75kEZAGPOl7WEn8RjOgAV1pImXyZnIvbic6WDynxCi6APz-E-f2OO8BjwhcSi39kr-XeyQhWnGzHdJTLkMXLzKDOyShl1CzmxJMRApq5tlt~v8S1iEFNJ~RgIL7A8ROrzNhDLCLveE19JYBjJt7hkosotgAaVa9vv8mKl-~K6GJE61pnY1jZZrZXVog0D8ZTTlywt6t1UEPc1Oy5sC-JGUEXQI8E8ziYeoBkaXjEK69TTP~4qyjgwxXYi56pnn7oiawkYtWXDNjmx2iA5DgfDs7-~8hS6yTGXo9siENRqRbHlCI-OZYHHuhZ3ffG5RAJ~GyqdQ__&Key-Pair-Id=APKAJLOHF5GGSLRBV4ZA.
Berger, Katja, Jochem Verrelst, Jean-Baptiste Féret, Tobias Hank, Matthias Wocher, Wolfram Mauser, and Gustau Camps-Valls. 2020. “Retrieval of Aboveground Crop Nitrogen Content with a Hybrid Machine Learning Method.” International Journal of Applied Earth Observation and Geoinformation 92 (October): 102174. https://doi.org/10.1016/j.jag.2020.102174.
Green, Robert O., B. E. Pavri, and T. G. Chrien. 2003. “On-Orbit Radiometric and Spectral Calibration Characteristics of EO-1 Hyperion Derived with an Underflight of AVIRIS and in Situ Measurements at Salar de Arizaro, Argentina.” IEEE Transactions on Geoscience and Remote Sensing 41 (6): 1194–1203. https://doi.org/10.1109/TGRS.2003.813204.
Kattge, Jens, S. Díaz, S. Lavorel, I. C. Prentice, P. Leadley, G. Bönisch, E. Garnier, et al. 2011. “TRY – a Global Database of Plant Traits.” Global Change Biology 17 (9): 2905–35. https://doi.org/10.1111/j.1365-2486.2011.02451.x.
Manifold, Bryce, Shuaiqian Men, Shuaiqian Men, Ruoqian Hu, and Dan Fu. 2021. “A Versatile Deep Learning Architecture for Classification and Label-Free Prediction of Hyperspectral Images.” Nature Machine Intelligence 3 (4): 306–15. https://doi.org/10.1038/s42256-021-00309-y.
Rasti, Behnood, Paul Scheunders, Pedram Ghamisi, Giorgio Licciardi, and Jocelyn Chanussot. 2018. “Noise Reduction in Hyperspectral Imagery: Overview and Application.” Remote Sensing 10 (3): 482. https://doi.org/10.3390/rs10030482.
Violle, Cyrille, Marie-Laure Navas, Denis Vile, Elena Kazakou, Claire Fortunel, Irène Hummel, and Eric Garnier. 2007. “Let the Concept of Trait Be Functional!” Oikos 116 (5): 882–92. https://doi.org/10.1111/j.0030-1299.2007.15559.x.
Ansprechpartner
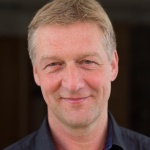
Norbert Haala
apl. Prof. Dr.-Ing.Stellvertretender Institutsleiter