Yiming Du
Towards Combining Volunteered Geographical Information and Remote Sensing for Local Climate Zone Classification
Duration of the Thesis: 6 months
Completion: July 2019
Supervisor: Dr.-Ing. Volker Walter
Examiner: Prof. Dr.-Ing. Uwe Sörgel
Introduction
Land cover is a fast growing research topic emerging with the development of remote sensing technology. In recent years, the concept of LCZ has been proposed, which provides us with a new way to describe land use/land cover. The scheme consists of 10 "built" classes and 7 "natural" classes. Nevertheless, the use of remote sensing technology alone to promote LCZ for cities around the world still faces great challenges. In recent years, the integration of high resolution remote sensing images and OSM in land cover classification has attracted growing number of attention [1]. Because of its high accuracy and integrity, some OSM data could directly superimposed on the classification map. For high spatial resolution remote sensing images, a lot of structure and detailed information can be then obtained [2].
Data Preprocessing
We manually label the 17 sub-class AOI of Stuttgart according to the WUDAPT standard process, However, in the actual annotation, we found that due to the urban characteristics of Stuttgart, the city did not have the class1 (compact high rise) and class7 (lightweight low rise). So in the end we labeled 15 sub-classes in the target area. In order to make the experimental results more reasonable, we chose the same data from Berlin's standard data. The data source comes from the standard data published by the 2017 IEEE GRSS Data Fusion Contest.
Methodology
This thesis proposes a method combining OSM morphological features with multispectral satellite images. In this master thesis, we chose four features in OSM data: buildings, roads, railways and waterways as input feature spaces, since these four features have the highest correlation with the sub-classes of LCZ [3][4]. We would extract discriminating spatial features from OSM based raster data by four operators taken from the mathematical morphological feature extraction theory. Considering the different characteristics of polygon and polyline vector OSM layers, we used different structure elements (SE), for example: square SE with kernel size of 3 for polygon data and disk SE considering 6 direction with kernel size of 1 for polyline data. and employs machine learning classifier (Random Forests) to obtain the final classification maps. The workflow of our proposed method is shown in the Figure 2.
Results
The experimental results show that the proportion of training data for each city to obtain the best accuracy results is different. But the same thing is that compared with using satellite image alone to do LCZ classification, theaddition of OSM features has an improvement of 1.5% - 2% for the final LCZ classification accuracy of different cities.
Table 1: Classification Performances for Different Random Forest Trees in Stuttgart
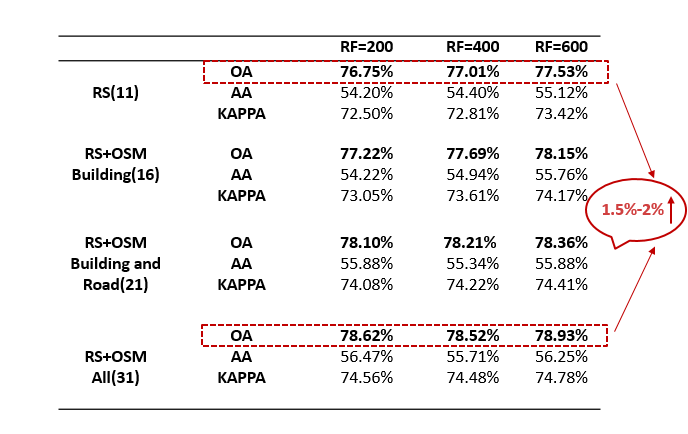
Table 2: Classification Performances for Different Random Forest Trees in Berlin
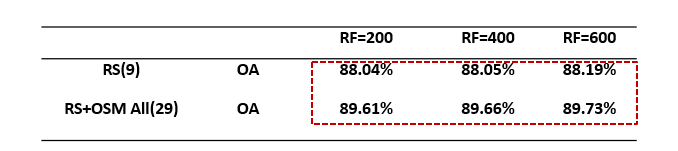
Conclusion
Experiments results confirms that VGI data, especially OSM vector data could provide significant improvement in the generation of LCZs, which is robust with respect to different variables (Tree numbers and Training Ratios).The most contribution of OSM data refers to road networks, while the second one is buildings. Waterways and Railways lead to less increase even decrease sometimes. (The curse of dimensionality [5])
References (selection)
[1]Fonte, Cidália C. et al. (2019). “Using OpenStreetMap (OSM) to enhance the classification of local climate zones in the framework of WUDAPT”. In: Urban Climate 28, p. 100456. ISSN : 2212-0955.
[2]Luo, Nianxue et al. (2019). “Fusing High-Spatial-Resolution Remotely Sensed Imagery and OpenStreetMap Data for Land Cover Classification Over Urban Areas”. In: Remote Sensing 11.1. ISSN : 2072-4292.
[3]Lopes, P. et al. (2017). “Using OpenStreetMap data to assist in the creation of LCZ maps”. In: pp. 1–4.
[4]Yokoya, N. et al. (2018). “Open Data for Global Multimodal Land Use Classification: Outcome of the 2017 IEEE GRSS Data Fusion Contest”. In: IEEE Journal of Selected Topics in Applied Earth Observations and Remote Sensing 11.5, pp. 1363–1377. ISSN: 1939-1404.
[5] Hughes, G.F. (January 1968). "On the mean accuracy of statistical pattern recognizers". IEEE Transactions on Information Theory. 14 (1): 55–63.
Ansprechpartner
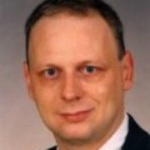
Volker Walter
Dr.-Ing.Gruppenleiter Geoinformatik