Yao Wang
Adersarial Network in Domain Adaption for Remote Sensing Image Classification
Duration of the Thesis: 6 months
Completion: January 2019
Supervisor: Prof. Dr. Xiaoxiang Zhu
Examiner: Prof. Dr.-Ing. Uwe Sörgel
With the increase of spatial and spectral resolution of remote sensing data, several researches and studies about classification are carried out with quite remarkable performances. However, as remote sensing data acquisition is affected by a lot of factors such as geographical location and time, the previous trained classifier will not behave well on the new data, especially the data from another region. Apart from this ‘data shift’, getting accurate labeled data or ground truth is very difficult and time-consuming. Therefore, it is essential to make full use of existed annotated data and well-trained model and applied them in other expanded datasets or areas. Lately, domain adaptation is brought in remote sensing to solve these obstructive problems for classification.
Inspired by the method of Adversarial Discriminative Domain Adaptation (ADDA) proposed by Tzeng et al. [1], we try to implement this method in remote sensing classification to solve the existed problem that training and test data distribution have distinct difference for the model. The advantage of ADDA for classification is that we can train an end-to-end CNN model for unsupervised domain adaptation. The procedure of ADDA for remote sensing image classification can be divided into three stages as shown in figure 1. Firstly, we need to pretrain CNN encoder and classifier with limited labeled source data. Then, take source CNN encoder as one of the inputs of discriminator network and use them to train target encoder. Finally, we can make use of the trained target CNN encoder and classifier to predict labels of target data.
We apply Adversarial Discriminative Domain Adaptation (ADDA) on remote sensing to investigate the performances on three dataset pairs, Berlin-Munich, London-Madrid and Pavia University-Pavia Centre. In each dataset pair, there are two experimental datasets called source domain and target domain separately. Our objective is to give predictions of target data with the help of limited source labeled data. For instance, Berlin is taken as source and Munich is target domain. We use limited labeled data on Berlin to pretrain classification model and it on the whole Berlin image for visualization shown in figure 2.
With available source CNN model, we can train target representation and discriminator by ADDA. To evaluate the performance of ADDA, we compare it with so-called Source Only which transfers source encoder on target data directly without adaptation. ADDA improves the accuracy of Source Only from 63.8% to 76.7% on Munich test data (Table 1). It means that ADDA adapts source CNN model on target so that it can fit better to different data. To have an intuitive classification result, we apply trained model under different methods on the whole target image Munich for visualization in figure 3. Although labels of target data are provided for adapted classification model testing that we will not need in our ADDA experiment, we display ground truth samples on Munich Sentinel-2 only to set a standard for comparison. We find that ADDA can show the complexity of land cover distribution in Munich. Moreover, it matches ground truth better than Source Only because that there should not be so many large low-rise buildings in the center of Munich.
Table 1: Characteristics and classification accuracies of Berlin and Munich
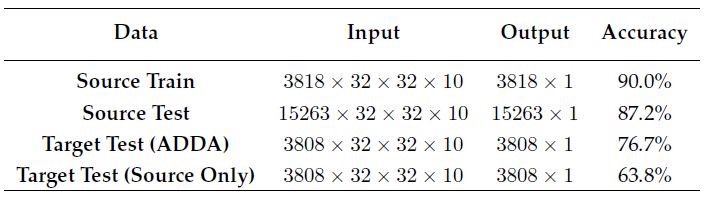
As a typical deep domain adaptation method that is a new kind of approaches with good performance in domain adaptation field, ADDA will be compared with some traditional algorithms to analysis its validity. Previous methods are always combined with some traditional classifier like SVM. In developing a successful SVM, the first step is feature extraction. Principal Component Analysis (PCA) and Kernel Principal Component Analysis (KPCA) are commonly used dimension-reduction approaches that can reduce a large set of variables to a small set, but still containing most of the information in the large set. In domain adaptation, we extract principal components of combined spaces of source and target domain. Then we take the projected variables as input of SVM to train the classifier. PCA-SVM and KPCA-SVM are the traditional domain adaptation methods that we will compare with ADDA and Source Only in Table 2.
Table 2: Accuracy evaluation indexes on Berlin and Munich
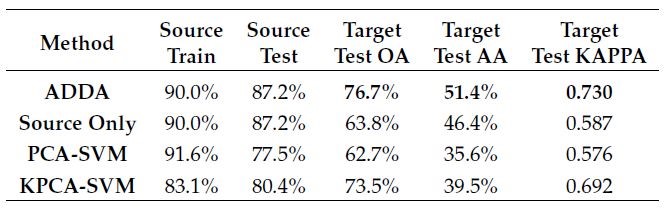
We analysis the results from different perspectives such as accuracy, evaluation metrics, and visual classification map. ADDA has showed reliable and stable performance on all dataset pairs. The result shows that ADDA performs much better compare to other methods.
Reference
1] Tzeng E., Hoffman J., Saenko K., and Darrell T. (2017). Adversarial Discriminative Domain Adaptation.
Ansprechpartner
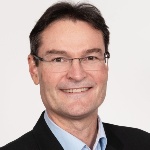
Uwe Sörgel
Prof. Dr.-Ing.Institutsleiter, Fachstudienberater