Marinos Vlachos
Integration and Testing of Sequoia MS Camera with Various UAV Platforms
Duration of the Thesis: 6 months
Completion: December 2017
Supervisor: Dr.-Ing. Michael Cramer
Examiner: Prof. Dr.-Ing. Norbert Haala
Introduction
Current high spatial resolution satellite sensors lack the spectral resolution required for many quantitative remote sensing applications and, given the limited spectral resolution, they only allow the calculation of a limited number of vegetation indices and remote sensing products. Additionally if short revisit time is required, the cost of high resolution imagery becomes quite larger thus is a limiting factor whereas sensors with shorter revisit time lack the necessary spatial resolution (Berni et al. n.d.). Combining high spatial resolution with short revisit times is crucial in order to generate useful remote sensing products related to agricultural and environmental applications. Alternatives based on traditional airborne platforms provide high spatial resolution and potentially short revisit time, but their use is limited by their high operational complexity and costs (Berni et al. n.d.).
In recent years an additional option has emerged for the aforementioned applications as well as traditional photogrammetric procedures. The standalone UAVs, combined with modern photogrammetric software, are enhanced by artificial vision algorithms, and are beginning to prevail, as they are inexpensive, easy to use, require minimal training and have many applications like traditional photogrammetric procedure or remote sensing applications regarding agriculture and environment (Nex and Remondino 2013).
The main developments leading to the rapid development of autonomous UAV in recent years are the incorporation of low cost GPS & INS for real-time navigation and cost reduction. The first reduces the need for an experienced pilot, allows navigation beyond the user's field of view, automatic flight planning, and recording of projection centers of images during flight (Skarlatos and Vlachos 2015).
Based on the above, recently a few imaging platforms have emerged into the market that are suitable for integration and operation with various UAV platforms and can provide high spatial resolution and short revisit time in order to fulfill the requirements of several remote sensing applications. The scope of this Master thesis is focused on the integration and testing of a specific MS camera system which is the Parrot Sequoia with various UAV systems that are either commercial like DJI Phantom 4 or custom built.
Methodology
Following the above, various inspections on the results as well as comparisons of the photogrammetric derived products were made in order to evaluate the capabilities of the Sequoia MS Camera system regarding the standard photogrammetric process in contrast with the results that a high resolution RGB camera system like Ricoh GXR Mount A12 with a ZEISS Biogon length of 21mm focal length (RicohZEISS) provided for the same areas.
The photogrammetric empirical testing was done in two Test Sites were the Sequoia MS/Custom copter system was used due to the capabilities of mounting multiple camera systems and operating them simultaneously as well as being able to power up the Sequoia system itself.
Hardware Integration
Sequoia features 3 sensors:
The multispectral sensor containing 4 separate arrays (Green, Red, Red Edge, NIR) 1,2MPix each, an RGB 16 MPix camera sensor and the sunshine sensor that records the intensity of light emitting from the sun in the 4 bands and later on these values can be used for radiometric correction on the images. The system incorporates GPS and IMU for increased accuracy of the collected data. The triggering is done through a web interface with a smartphone using WiFi.
A manual flight was done with the Sequoia/DJI Phantom 4 system in order to evaluate the functionality of the integration but no photogrammetric empirical testing was done using that particular system.
Results
In this section the results from the bundle block adjustment and the derived products related to Remote Sensing and Photogrammetry are presented for 1 of the 4 blocks captured by Sequoia. Additionally a comparison is made between the photogrammetric products that the Sequoia MS and Ricoh ZEISS RGB camera systems provided
Flights overview
In Table 1 the details regarding the block captured with the Sequoia MS camera and the block captured with Ricoh GXR Mount A12 with a ZEISS Biogon length of 21mm focal length during the same flight as they were operated simultaneously.
Table 1: Blocks Overview
Vegetation Site Flight 65m Sequoia |
Vegetation Site Flight 65m RicohZEISS |
|
---|---|---|
Overlap |
80% |
80% |
No. of Images |
780 |
213 |
Flying Height |
65m |
65m |
GSD |
6,95cm |
1,77cm |
no #GCP |
9 |
5 |
Bundle Adjustment Overview
RMS errors as well Standard deviation values in all 3 directions are very small, varying up to 30% of the GSD
Inspection of Photogrammetric derived products from Sequoia MS
Feature matching and object reconstruction was successful for the majority of the block. The DSM describes the outlines and the shape of trees without the presence of noise. The same can be said for the orthophotos as no distortions are present. There are some blind spots at the edges of the block (line 3) due to the lack of sufficient number of images.
Accuracy Evaluation of the DSMs Derived by the 2 camera systems
In order to further evaluate which DSM provided the most accurate results thus which camera system is indicated to be more accurate overall, a statistic analysis was made where the height differences between the measured 3D points derived DSMs were evaluated and compared as is shown in the table below.
Table 2: Statistics of the height differences between the 3D points and DSMs height differences for the Vegetation Monitoring test field 65m block
Ricoh_diff (m) |
Sequoia_diff (m) |
|
---|---|---|
Mean (m) |
0,0786 |
0,2678 |
StdDev (m) |
0,0291 |
0,0344 |
RMS (m) |
0,0833 |
0,2697 |
The standard deviations values are at 1,7*GSD (GSD=1,77cm/pixel) and 0,5*GSD(GSD=6,95cm/pixel) of the Images respectively. The RMS error values though are significantly larger than the standard deviations close to 3*Std for the RicohZEISS DSM and 8,5*Std for the Sequoia MS DSM. That is due to some systematic errors in the heights of the signalized 3D points which is indicated by the fact that all the height differences in the 2 comparisons are positive as is shown in Figure 5.
Derived Products related to Remote Sensing
Using Pix4D Mapper maps referring to reflectance and indices like NDVI were extracted. In order to do that a radiometric calibration was done in the images using images of a radiometric target (X-Rite ColorChecker®) in order to radiometrically calibrate the blocks.
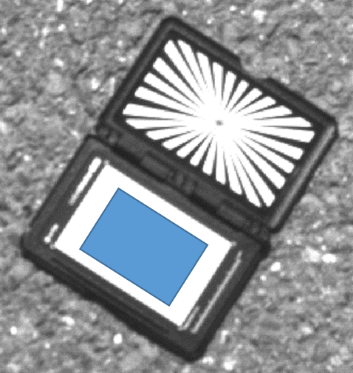
Figure 6: Drawn polygon inside the target in order to estimate the difference between the reference value and the value in the pixels (in this case was done for the Green Band)
Below a Reflectance map referring to the green band as well as an NDVI map are presented below.
Conclusion
The Integration of Sequoia with the 2 UAV platforms was successful as it’s indicated by the final results. Mini MS cameras like Sequoia can minimize the number of non-matched images in the areas where vegetation is present. Sequoia MS Camera/Custom Copter system maximized the feature matching between the images.
The system can be used as a photogrammetric platform as the Bundle adjustment results for the test field blocks showed. There were no errors bigger than 30% of the GSD due to the better point distribution regarding the 3D network and due to the Sequoia MS system itself as it proved that MS Imagery can deal more sufficiently with the problems of feature point matching in vegetation areas. The capabilities of the system regarding various Remote Sensing applications were not investigated during this master thesis. Future research using the derived RS products can indicate if the use of small MS aerial platforms like the Custom copter/Sequoia and the results they provide, is more beneficial
References (selection)
Berni, J. A. J., Zarco-tejada, P. J., Suárez, L., González-dugo, V., and Fereres, E. (n.d.). “REMOTE SENSING OF VEGETATION FROM UAV PLATFORMS USING LIGHTWEIGHT MULTISPECTRAL AND THERMAL IMAGING SENSORS.”
Nex, F., and Remondino, F. (2013). “UAV for 3D mapping applications: a review.”
Skarlatos, D., and Vlachos, M. (2015). “Investigating influence of UAV flight patterns in multi-stereo view DSM accuracy.”
Ansprechpartner
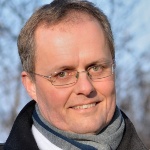
Michael Cramer
Dr.-Ing.Gruppenleiter Photogrammetrische Systeme