Xinjie Huang
PCA-based Change Detection Using Multi-temporal SAR Images
Duration of the Thesis: 6 months
Completion: August 2017
Supervisor: Chia-Hsiang Yang
Examiner & supervisor: Prof. Dr.-Ing. Uwe Sörgel
Introduction
Due to the rise in population and rapid rate of city expansions, vast infrastructure development and building construction occurs (C. H. Yang & Soergel 2016). Urban changes have received a great deal of attention recently. To measure and document these changes provides a foundation for a large number of applications, such as local, regional management, urban change monitoring, urban planning, and environmental studies (Kamusoko et al. 2013, Marin et al. 2015).
In contrast to conventional field surveys, which are expensive and timeinefficient, remote sensing provides a cost-effective and efficient alternative to the ground-based survey in mapping and monitoring environmental changes in general and urban changes in particular. Compared with other remote sensing techniques, Synthetic Aperture Radar (SAR) is independent of solar illustration and weather conditions. Also it is capable of covering vast areas in short time with considerable fine spatial resolution. These capabilities make it suitable for monitoring and change detection tasks.
To monitor the urban changes, it is of great significance to gain the change information of both temporal variation and spatial features. Principal Component Analysis (PCA) is a very powerful statistical analysis method, which is capable of decomposing data in terms of maximal variance and obtaining uncorrelated components in spatial and temporal domain (Jianqin et al. 2010, Singh et al. 2004). We then proposed a PCA-based method for multi-temporal change detection.
In this thesis, to monitor the characteristic of the changes in urban area, PCA is applied to time series of TerraSAR-X images. A case study in Berlin, Germany is selected for construction monitoring. The temporal and spatial patterns of changes in target area are derived from the image time series using PCA. We then extract the ground changes through a thresholding process. After validation against ground truth provided by Google Earth, the results represent good consistency.
Methodology
As we know, in this study, we use n images at different time epochs. Thus, the data matrix Xn×l×m is three dimensional, where n is the number of images, l,m refer to the size of images. In order to apply PCA later on the data, we simply rearrange the data sets into two-dimensional matrix Xn×p, in which p denotes the number of pixels on each image.
Consequently, to extract the change parts in each image, we apply a thresholding process on those selected PCs. The procedure is illustrated in the flowchart in Figure 2.
Results
For the visualization purpose as well as for testing the reliability of our method, ground truth from Google Earth and the PCA results of a smaller patch are combined, giving a credible proof to this method. This patch is a businiess distric near the city center. Buildings change frequently duiring the study period. Changed objects are highlighted by red polygons in Figure 3.
Conclusions
Based on the work we have done in the thesis, the following conclusion would be drawn as below:
- Our method has the capability of extracting spatio-temporal changes from satellite time series images. The results have evidently proved the performance of our PCA-based change detection method using multi-temporal SAR images.
- Building demolition and erection as well as renovation can be distinctly recognized along with their change times. As we illustrate in Chapter 4.5 and Chapter 4.6, changes are successfully detected, along with their times.
- Our method can extract the significant information by maximum variance from data set, while reducing unimportant information like noise. This advantage makes our technique suitable for high-resolution image data analysis, which has been tested by TerraSAR-X images in this study.
- We select temporal patterns resulted from PCA decomposition only by priori knowledge and experience, i.e. certain types of temporal patterns are regarded as indication of building change. This probably leads to missing selection of patterns.
References
C. H. Yang, B. K. K. & Soergel, U. (2016), 4d change detection based on persistent scatterer interferometry, in ‘2016 9th IAPRWorkshop on Pattern Recogniton in Remote Sensing (PRRS)’.
Jianqin, M., Jingjing, G. & Xiaojie, L. (2010), ‘Water quality evaluation model based on principal component analysis and information entropy: Application in jinshui river’, Journal of Resources and Ecology 1(3), 249–252. URL: http://www.bioone.org/doi/abs/10.3969/j.issn.1674-764x.2010.03.008
Kamusoko, C., Gamba, J. & Murakami, H. (2013), ‘Monitoring urban spatial growth in harare metropolitan province, zimbabwe’, Advances in Remote Sensing 2(04), 322.
Marin, C., Bovolo, F. & Bruzzone, L. (2015), ‘Building change detection in multitemporal very high resolution sar images’, IEEE Transactions on Geoscience and Remote Sensing 53(5), 2664–2682.
Singh, K. P., Malik, A., Mohan, D. & Sinha, S. (2004), ‘Multivariate statistical techniques for the evaluation of spatial and temporal variations in water quality of gomti river (india)—a case study’, Water Research 38(18), 3980 – 3992.
URL: http://www.sciencedirect.com/science/article/pii/S0043135404003367
Ansprechpartner
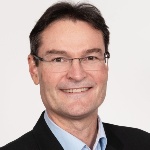
Uwe Sörgel
Prof. Dr.-Ing.Institutsleiter, Fachstudienberater