Xiaofeng Zhang
Dynamic Objects Classification Based on High Resolution Doppler Radar and Laser Data
Duration of the Thesis: 6 months
Completion: January 2017
Supervisor: Dipl.-Ing Jakob Lombacher, M.Sc. Peter Broβeit, Daimler AG
Examiner: Prof. Dr.-Ing. Norbert Haala
Introduction and Motivation
As the development of urbanization and modern mobility, the number of private cars has been growing rapidly year by year, especially in developing countries. As the prediction in [Mat06], the deaths caused by traffic accident will reach 2.1 million in 2030, which make road safety an unavoidable topic in the development of modern transportation.
The research recent years in the fields of Advanced Driver Assistant System (ADAS) and Autonomous Driving System, gives us the promising vision of taking our hands off the wheel while providing safer driving experiences. As a foundation of autonomous driving system and a major part of environment perception algorithm, a feature based road dynamic object classification system is designed and studied in this thesis. Based the sensor data collected during the test drive, the designed dynamic classification system shall be able to classify the moving objects into one of the four following classes: vehicle, bicyclist, pedestrian, pedestrian group.
Contribution
The training process is used to train the classifier, while the classification process is used to test the classification results.
Training process: Training data is the input data for the training process. It consists of labelled radar data, and laser sensor data. Then the input data are prepared in data preprocessing step and dynamic clusters are extracted as the basic units for feature extraction. Related data preprocessing methods like data association for sensor data are studied. A number of features are investigated and extracted based on the characteristics of road users. In total 35 radar features and 25 laser features are designed based on the characteristics of the dynamic road users. Important features for classification are selected and investigated under different circumstances and restrictions. Hyper-parameters for learning models are also fine-tuned during the training process. The output of the training process the classifier for both Support Vector Machine (SVM) and AdaBoost [Dud12].
Classification process: The test data consist of pre-clustered radar data and real-time laser sensor data. Then the test data are preprocessed and the selected features are extracted individually from the laser cluster and radar cluster. Based on the extracted features and trained classifier model, the corresponding classification results are evaluated and compared between different classification methods.
3 Conclusion
The evaluations were done using real world data, and an overall good classification accuracy An overall 98.97% classification accuracy based on both sensor data are obtained for Support Vector Machine classifier and 97.95% accuracy is obtained for AdaBoost classifier) is obtained. Selected interesting features for both sensor and evaluation results under different constrains are also discussed in this thesis.
The designed system shows a promising classification rate for dynamic road users despite of the possible noises. The system performance can be improved by further investigation into the preprocessing stage like clustering method and more precise data association method. Different classification method like neural network could also be used in future work.
References:
[Mat06] Mathers, C. D., & Loncar, D. (2006). Projections of global mortality and burden of disease from 2002 to 2030. Plos med, 3(11), e442.
[Dud12] Duda, R. O., Hart, P. E., & Stork, D. G. (2012). Pattern classification. John Wiley & Sons.
Ansprechpartner
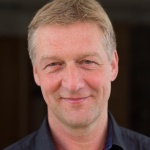
Norbert Haala
apl. Prof. Dr.-Ing.Stellvertretender Institutsleiter